Manage OneDrive settings on macOS using property list (Plist) files. After the OneDrive sync app for Mac is installed, users can configure settings for the app. These settings are called preferences. As an administrator, you might want to provide users in your organization with a standard set of preferences. Some apps will update their.plist file every time you use the program. Otherwise, open the app and make any type of change in its preferences and save. Go back to the Preferences window and see which.plist file jumps to the top of the list. kirkmc adds: Good common sense. I've used this many times over the years, and I couldn't find it in. In a previous post, I discussed how to create an XML file to report on custom inventory. For simple custom data objects, the approach described in that post works fine, however, if you need to send up multiple inventory objects to the same inventory key and if you need the inventory to be reported into a location other than the Custom Data inventory tree; including placing the data into.
Source code:Lib/plistlib.py
This module provides an interface for reading and writing the “property list”files used by Apple, primarily on macOS and iOS. This module supports both binaryand XML plist files.
The property list (.plist
) file format is a simple serialization supportingbasic object types, like dictionaries, lists, numbers and strings. Usually thetop level object is a dictionary.
To write out and to parse a plist file, use the dump()
andload()
functions.
To work with plist data in bytes objects, use dumps()
and loads()
.
Values can be strings, integers, floats, booleans, tuples, lists, dictionaries(but only with string keys), bytes
, bytearray
or datetime.datetime
objects.
Changed in version 3.4: New API, old API deprecated. Support for binary format plists added.
Changed in version 3.8: Support added for reading and writing UID
tokens in binary plists as usedby NSKeyedArchiver and NSKeyedUnarchiver.
See also
Apple’s documentation of the file format.
This module defines the following functions:
plistlib.
load
(fp, *, fmt=None, dict_type=dict)¶Read a plist file. fp should be a readable and binary file object.Return the unpacked root object (which usually is adictionary).
The fmt is the format of the file and the following values are valid:
None
: Autodetect the file formatFMT_XML
: XML file formatFMT_BINARY
: Binary plist format
The dict_type is the type used for dictionaries that are read from theplist file.
XML data for the FMT_XML
format is parsed using the Expat parserfrom xml.parsers.expat
– see its documentation for possibleexceptions on ill-formed XML. Unknown elements will simply be ignoredby the plist parser.
The parser for the binary format raises InvalidFileException
when the file cannot be parsed.
plistlib.
loads
(data, *, fmt=None, dict_type=dict)¶Load a plist from a bytes object. See load()
for an explanation ofthe keyword arguments.
New in version 3.4.
plistlib.
dump
(value, fp, *, fmt=FMT_XML, sort_keys=True, skipkeys=False)¶Write value to a plist file. Fp should be a writable, binaryfile object.
The fmt argument specifies the format of the plist file and can beone of the following values:
FMT_XML
: XML formatted plist fileFMT_BINARY
: Binary formatted plist file
When sort_keys is true (the default) the keys for dictionaries will bewritten to the plist in sorted order, otherwise they will be written inthe iteration order of the dictionary.
When skipkeys is false (the default) the function raises TypeError
when a key of a dictionary is not a string, otherwise such keys are skipped.
A TypeError
will be raised if the object is of an unsupported type ora container that contains objects of unsupported types.
An OverflowError
will be raised for integer values that cannotbe represented in (binary) plist files.
plistlib.
dumps
(value, *, fmt=FMT_XML, sort_keys=True, skipkeys=False)¶Return value as a plist-formatted bytes object. Seethe documentation for dump()
for an explanation of the keywordarguments of this function.
New in version 3.4.
The following classes are available:
plistlib.
UID
(data)¶Wraps an int
. This is used when reading or writing NSKeyedArchiverencoded data, which contains UID (see PList manual).
It has one attribute, data
, which can be used to retrieve the int valueof the UID. data
must be in the range 0 <= data < 2**64.
The following constants are available:
plistlib.
FMT_XML
¶The XML format for plist files.
New in version 3.4.
plistlib.
FMT_BINARY
¶The binary format for plist files
Examples¶
Generating a plist:
Parsing a plist:
Since version 10.5 “Leopard”, Mac OS X has had DTrace, a tool used for performance analysis and troubleshooting. It provides data for Apple’s Instruments tool, as well as a collection of command line tools that are implemented as DTrace scripts. I’m familiar with the latter as I wrote the originals for the DTraceToolkit, which Apple then customized and enhanced for Mac OS X where they are shipped by default (great!). I use them regularly to answer this question:
why is my MacBook slow?
I work in an office where everyone has MacBook Pros, and “why is my MacBook slow?” is a common question. Applications can become slow or unresponsive while waiting for CPU work, memory requests or disk I/O to complete.
For people who try to ignore the slowdown, the question can become:
why is my MacBook fan so loud?
Standard performance analysis tools like Activity Monitor and top(1) (and any third-party tools based on the same foundation) can’t tell you some key information about activity on your system, such as how much CPU consumption is caused by short-lived processes, or which processes are causing disk I/O. DTrace, however, can see (just about) everything.
In this post, I’ll cover the top ten Mac OS X DTrace scripts that I use for figuring out why laptops are slow or why applications are misbehaving. Most of these scripts are already installed, a few are from the new DTrace book.
How to use DTrace
If you’ve never run a DTrace script before or even used the command line, here’s a basic walkthrough:
Open Terminal
Run the “Terminal” application. You can find it here in Finder:
You can also type “terminal” in Spotlight (the magnifying glass in the top right corner of your Mac’s screen), which should find it. I usually drag it to my Dock so it’s easy to find later:
Customize Terminal
When you first run Terminal, it’ll probably look like this:
I find the default font small and hard to read. The size of the window in terms of characters is also small (80 columns by 24 rows), presumably to pay homage to original Unix terminals of that size. (Why Unix? Mac OS X is Unix under the hood: the Darwin kernel).
You can adjust the font to your liking in Terminal->Preferences->Text. I use Monaco 13pt, with “Antialias Text” on. Under the “Window” tab is the default size, 80×24, which you can also increase later by clicking and dragging the bottom right corner of the terminal. For example, here’s my screen as I write this blog post (in a terminal-based text editor).
Running top
While DTrace can see everything, there are some things already covered by easy-to-use (and easy-to-type) tools, like top(1). Now that you have Terminal running, type “top -o cpu” and hit enter, which will refresh the screen showing top running processes. Type “q” to exit the top program. The output will look something like this:
I’ve truncated the header block to just include the columns. Look at the “%CPU” column to see which processes are making the CPUs busy, and the “RSIZE” column to see who is consuming main memory. The busiest (CPU) process will be at the top, since we sorted on cpu (“-o cpu”). The top was “firefox-bin” (Mozilla Firefox) at 98.8% CPU, which is in terms of a single processor (this has two). If firefox stayed that high you could look for the responsible tab and close it down, or restart Firefox.
After top, I turn to DTrace.
Running DTrace
DTrace requires admin privileges, so to use it you’ll usually need to type in a password to authenticate, provided you have administrator access (if you aren’t sure you do, click here to see how to check).
You can run DTrace by prefixing your DTrace commands with “sudo”, which will prompt for the password the first time around (but not for some time after that). When a DTrace command is running, you usually type Ctrl-C to end it. Here’s an example:
If sudo says “permission denied”, check your admin status.
and here’s what happens if you forgot the “sudo”:
Scripts
The scripts follow. A note on style: if the script ends with “.d”, it’s a basic DTrace script. If it doesn’t, then it’s a shell-wrapped script that provides command line options. Use “-h” to list them.
And if you’d like to learn DTrace, I’d recommend starting by reading the basic ones.
1. iosnoop
This “traces” disk I/O execution live. Each time a disk I/O completes, a line of output is printed to summarize it, including process name and filename details:
This lets you instantly find out which applications are using the disk, and what files they are reading or writing to. Disk I/O is typically slow (for non-SSD disks), so an application calling frequent disk I/O (a dozen per second or more) may run slowly as it waits for the disk I/O to complete.
The output columns show: UID = user ID, PID = process ID (unique identifier for the process), D = direction (R = read, W = write), BLOCK = location on disk, SIZE = I/O size in bytes, COMM = process name, PATHNAME = trailing portion of file pathname.
In that output I caught Google Chrome reading from a cache file (“data_2″), and writing to cookie files (“Cookies-journal” and “Cookies”). TweetDeck also wrote to database files (“td_26_brendangregg.db” and “td_26_brendangregg.db-journal”). The “??” is where the path information ends for iosnoop (if you are a darwin programmer and want to take a swing at improving that, see the fi_pathname translator in /usr/lib/dtrace/io.d).
The “-h” option lists options (don’t need “sudo” for this):
For tricky performance issues I often use “-stoD” to get start and end timestamps for each I/O in microseconds, and a couple of different types of I/O time calculations.
In hindsight, I should have called it diskiosnoop, since “io” could refer to different locations in the kernel I/O stack.
2. hfsslower.d
This script answers an iosnoop FAQ: why dosen’t iosnoop see my application disk I/O?
The reason is that applications rarely request disk I/O directly, rather, they access a file system that does disk I/O on their behalf. To increase performance, the file system will usually try to cache as much file data as possible in main memory (DRAM). The application may (by some notion) think that it’s doing disk I/O, but it’s actually reading from very fast DRAM, thanks to the file system. Writes can also buffer in DRAM and write to disk later, which also speeds up application performance.
The hfsslower.d script measures I/O before it is processed by the HFS+ file system (Apple’s current default file system). iosnoop measures I/O after the file system, and only if it reaches disk:
A couple of points:
- hfsslower.d will see a lot more I/O than iosnoop, as it includes file system cache hits.
- hfsslower.d better reflects application performance, as it measures the same latency that the application directly suffered.
The hfsslower.d script is from the DTrace book, and can be found here. To run it, you’ll need to create a text file containing the script (or pull it from the DTrace book tarball), and make the file executable from Terminal by running “chmod 755 hfsslower.d”.
This script takes an argument which is the minimum number of milliseconds to show I/O for. Here’s tracing 1ms HFS+ I/O and slower:
Columns are: TIME = time of I/O completion, PROCESS = application name, D = direction (R = read, W = write), KB = I/O size in Kbytes, ms = I/O latency in milliseconds, FILE = filename.
If you use the argument “0″, it will trace everything. If I’m chasing down slow I/O, I’ll often use an argument of “10″ for I/O slower than 10 milliseconds.
At this point you may think: if you just care about slow I/O, then just use iosnoop. That works to a point, but there can be slow I/O caused by something other than disks (file system lock contention, for example). The other advantage of the hfsslower.d script is that the measured latency matches the application pain suffered, whereas at the disk level you can only assume a correlation.
3. execsnoop
This traces the execution of new processes. This is great at identifying short-lived processes that may be caused by misbehaving applications and can slow down your system. These short-lived processes are usually too quick to be picked up by standard monitoring tools like the Activity Monitor or top(1).
To demonstrate this tool, here’s what happens when you type “man ls”:
This prints a line for each new process that is executed, in a rolling output similar to the previous tools. The fields are: STRTIME = (string) timestamp, UID = user ID, PID = process ID, PPID = parent process ID, ARGS = process name (should be process + arguments, but that doesn’t yet work on Mac OS X; if you want to debug, see pr_psargs in /usr/lib/dtrace/proc.d).
Here’s what happens when I turn AirPort (wifi) off, then on:
Near the end I opened up a new tab in Mozilla Firefox and another in Google Chrome. You can see a fundamental difference: Chrome creates a new process for that tab, Firefox doesn’t.
execsnoop has various options, use -h to list them all. I used -v above, to print the time.
4. opensnoop
This traces file opens and prints various details, including the time and error code when using “-ve”. I usually use it to look for failed opens, which can be a sign of misconfigured applications. Discovering their config files and resource files can also be useful.
While tracing, I changed the clock in the top bar from digital:
to analogue:
The files opened to performed this can be seen by the “SystemUIServer” process, beginning with a Preferences file and then some PDFs. Huh? PDFs? What are those PDFs?
That’s bizarre. I didn’t know that those tiny icons were implemented as PDFs!
5. dtruss
Script To Generate The Plist For Macbook Pro
The previous two tools, opensnoop and execsnoop, operate by tracing specific system calls. A system call (or “syscall”) is what an application performs to request the operating system kernel to perform privileged work, including process creation, file operations, and other I/O (eg, disk or network I/O). Syscalls are a great target for analysis with DTrace, since examining them often provides a pretty good picture of what an application is trying to do. They can also provide useful details including byte counts, file and process names, error codes, and latency.
The dtruss tool traces all types of system calls, which is very useful for general debugging, especially since Mac OS X doesn’t come with a standard syscall tracer (like Linux’s “strace” or Solaris’s “truss”). One advantage of dtruss over those other tools is that dtruss can trace multiple processes at the same time, matching on the process name “-n”. For example, tracing Firefox via its process name “firefox-bin”:
The output will be many pages, as applications commonly make frequent system calls.
dtruss can also launch and trace a program. Here the humble “ls -l” command was traced:
100 lines were trimmed to keep that example short.
dtruss supports various options, including the printing of system call timing for use when analyzing performance.
I’ve used the “-e” option many times to figure out what’s slowing down an application. It shows the elapsed time for the system call in microseconds:
I then look down the “ELAPSD” column for the largest times.
A dtruss FAQ is: why is the output in the wrong order?
As with other DTrace “tracing” style tools (that print lines of output as they occur) the output can be shuffled slightly due to CPU buffering on multi-CPU systems. The “-d” option for relative times (since program start) is useful for cases where the output order is important. You can then post-sort by that column so that the dtruss output is in the correct order. (For example, by redirecting the output to a file, then using the sort(1) command on that column.)
Ryan Dahl (creator of node.js) had been using dtruss so frequently recently that he made some enhancements and posted a newer version on github here.
6. soconnect_mac.d
This script is from the DTrace book, and traces outbound TCP connections along with details:
It’s a quick way to find out what applications are connecting to whom on the Internet. The script is here.
Columns are: PID = process ID, PROCESS = process name, FAM = protocol family (2 = IPv4), ADDRESS = IP address, PORT = TCP destination port, LAT(us) latency of the connect() system call in microseconds, RESULT = return of the connect().
Many connections these days are “non-blocking”, so the latency appears low and has the result “In progress”. The actual connection completes sometime later (the timing can be traced using some more DTrace, when desired).
There is a companion tool, soaccept_mac.d, which shows inbound TCP connections. If you ran both regularly, you may catch something performing networking that shouldn’t be (e.g., spyware, virus, …).
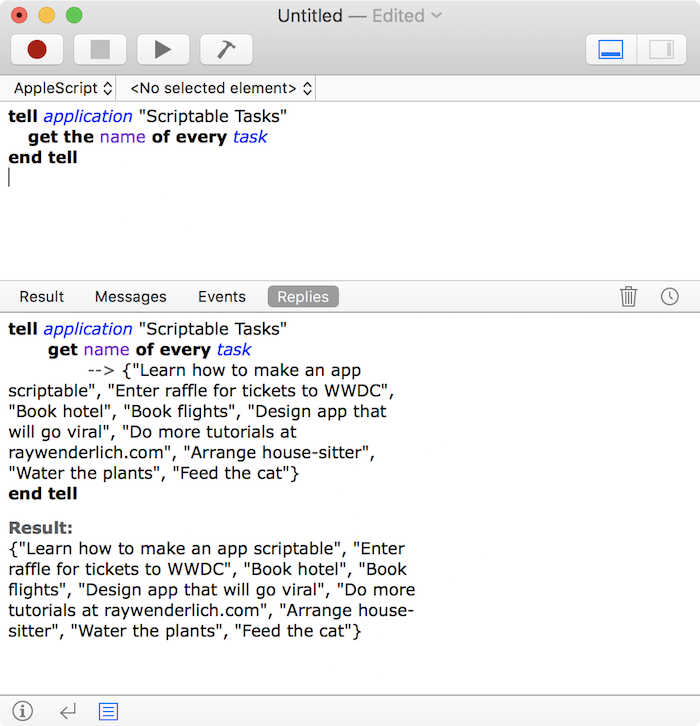
7. errinfo
This tool provides a summary of which system calls were failing, showing the process name, error code, and short description of the error:
This can be another quick way to track down failing or misconfigured applications. Note that (usually) most system call errors are not a problem: it can be normal for system calls to fail, and the calling application handles that condition correctly. errinfo is particularly useful when the application didn’t handle the failure correctly, and the system calls are silently failing.
8. bitesize.d
This is a simple DTrace script that characterizes the disk I/O workload, showing a distribution of the size of the I/O in bytes along with the application name:
The “value” column shows the minimum size of the I/O in bytes, and the “count” column shows the number of I/O in that range: between the minimum size and the next size shown.
To follow an example: the “bsdtar” command shown above performed 151 disk I/Os that were between 32768 bytes and 65535 bytes in size (32K to 64K), which was where most of its I/O fell. The text plot in the middle provides a visualization for the distribution of the events, which shows a spike that corresponds to this 32K to 64K range. Google Chrome did a couple of dozen I/O between 4 and 16 Kbytes.
Disk I/O is an expensive operation, so it’s usually preferable for it to be larger in size to improve the throughput to the device.
9. iotop
This presents the same data as iosnoop, but in a summarized way similar to top(1). It’s handy when disk I/O is so frequent that iosnoop is too verbose, and you want a high level summary of which process is rattling the disks. Here I’ve used “-CP” to not clear the screen and provide a rolling output (-C), and to show disk busy percentages (-P):
The “??” is for where some details aren’t yet available to DTrace, for the same reason as was described for the iosnoop script (if you are a programmer, you can see what needs to be fixed in the /usr/lib/dtrace/io.d file).
If you get “dynvardrops”, sorry: back when I wrote this (6 years ago), I never saw a disk workload heavy enough to cause these. A remedy can be to increase the dynvarsize tunable by adding the following line:
near this one:
in /usr/bin/iotop. You may want to make a backup of iotop first (iotop.orig) or copy it somewhere else to run it.
10. maclife.d
This script is from the DTrace book, and traces the creation and deletion of files:
Interesting! While tracing I saved the file I was editing in vim, which is seen in the last four lines. This tells me that vim is creating and removing temporary files as part of the save process.
Also note that it looks like TweetDeck created the file twice before removing it (two VNOP_CREATEs followed by a VNOP_REMOVE). This isn’t the correct order, which can be seen by examining the TIME(ms) column. A side-effect of DTrace’s negligible performance impact design is that output can be slightly shuffled due to the way it collects data from per-CPU buffers. I often include a TIME column like that one, not just for the usefulness of knowing time, but also as a means to post sort the output.
Other Scripts
There are other tools ready-to-go in Mac OS X. If you run the “man -k dtrace” command in a Terminal (or “apropos dtrace”), you’ll see the following output:
Plus more in the DTrace book.
And More…
DTrace isn’t just about running scripts; you can write your own custom scripts, run one-liners, and use higher-level tools that use DTrace behind the scenes, like Apple’s Instruments and Joyent’s Cloud Analytics. And it’s for more than just your MacBook: if you are using servers that have DTrace available, you can use it to diagnose their performance and issues too, including tracing kernel and application code. I regularly use it to see how a MySQL database interacts with a kernel file system, for example.
For more reading about DTrace, you can see my posts tagged dtrace, other blogs on dtrace.org, the original 410 page DTrace guide, 1100 page DTrace book, and my original DTrace page (which includes the DTraceToolkit). It’s a little old now (and I think prustat needs updating), but there’s also Matty’s Top Ten DTrace Scripts, which includes some of those above; and Greg Miller’s Exploring Leopard with DTrace, which includes Objective-C tracing.
For another post on Mac OS X performance, see my USE Method-based Mac OS X performance checklist.
In: DTrace · Tagged with: dtrace, macosx, top10